13.10.2023
The Impact of AI on Data Center Design
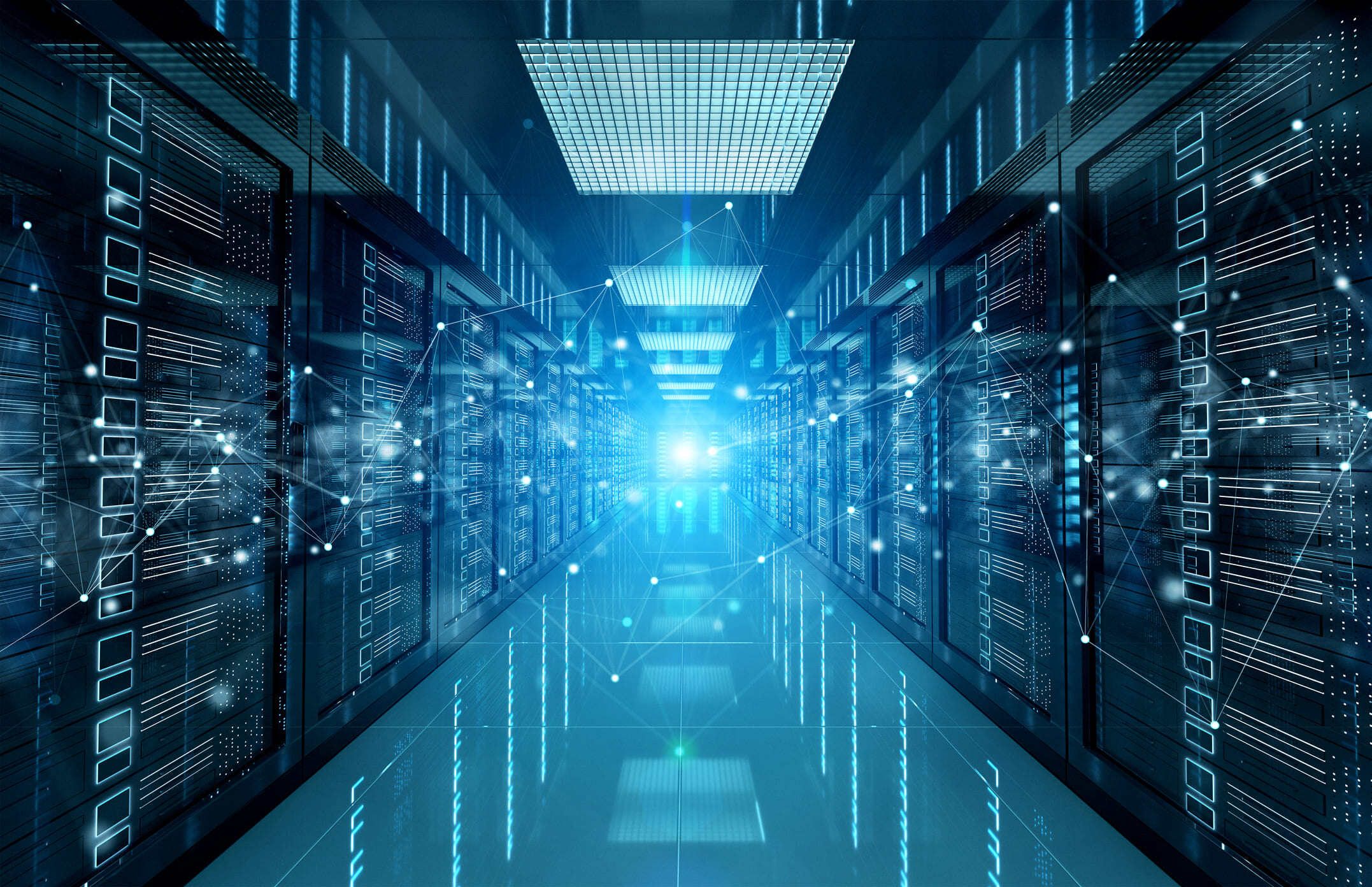
Designing a data center is a multifaceted challenge. It requires meticulous planning for power provision, cooling systems, robust security, consistent reliability, and lightning-fast network connectivity, all of which must harmoniously integrate. However, the rapid adoption of AI and machine learning models is reshaping data center design, driving unprecedented demand for power, cooling, and high-speed networking. AI workloads are far more compute-intensive than traditional applications, requiring entirely new infrastructure solutions to scale efficiently.
AI Training: Data Center Demands
AI models, like those used in medical and climate research or large language modelling or financial services, demand relentless computational power. Unlike some traditional data center workloads that experience peaks and troughs, AI model training is continuous. And the current trend of the latest AI algorithms is increasing the computational demands of AI enabled data centers.
Most conventional data centers are not equipped to deal with the enormous compute required to train the neural networks required of AI technology. Specialized infrastructure requirements to meet the updated demands of AI include:
- Higher-density racks: AI workloads require racks capable of supporting 40-60 kW per rack, a significant increase from traditional 5 kW racks.
- Advanced cooling solutions: The high heat output of AI workloads necessitates liquid cooling technologies, as traditional air cooling struggles to keep up.
- Optimized network architecture: AI training depends on high-bandwidth, low-latency connectivity, requiring specialized networking solutions like CXL and NVLink.
- Structural adaptations: AI computing cabinets are significantly heavier than conventional servers, requiring reinforced floors and rack systems to support loads exceeding 1.5 tonnes.
- Security and data protection: AI models are increasingly trained on proprietary datasets, requiring enhanced infrastructure security to protect data integrity.
Let’s take a look at each in more detail.
Increased Computational Requirements
First and foremost is the need for higher density racks. Whereas the average data center rack density a few years ago was 5 kilowatts (kW) per rack, the latest generation of AI supercomputers, like the NVIDIA DGX H100, support up to 40-60 kW per rack.
Just four of these systems in one rack could consume more than 40 kW while only occupying 60% of the space of a typical computing rack. While housing more computational power within a smaller space offers substantial cost-efficiency, it does present some unique challenges.
Implications for Power and Cooling
Traditionally cooled data centers have resorted to widely spaced racks to alleviate heat issues. But at-scale machine learning applications require server racks placed close together – as this optimizes the network configuration that is made up of very expensive high-throughput cables between servers, while also minimizing the overall cost of deployment.
This rising rack density in data centers is putting strains on conventional air cooling technologies. As a result, liquid cooling is gaining traction in AI data centers. It also places new emphasis on sustainable data center solutions, with providers who invest in sustainable practices offering future-proofed services to their customers.
The Role of High-Bandwidth Network Infrastructure in AI Data Centers
With faster and hotter servers, data centers must also adapt their network architecture and connectivity. Low-latency east-west spine and leaf networks are essential to support production traffic and machine learning processes. Additionally, investments in cache-capable systems like CXL and NVLink are necessary to ensure efficient data transfer between CPUs, GPUs, and DPUs without causing delays.
Structural Considerations for AI-Optimized Data Centers
AI-optimized data centers must also be structurally robust. These data centers need to support the transportation and installation of exceptionally heavy AI computing cabinets, some weighing over 1.5 tonnes or more when fully configured. This structural integrity is essential for the efficient operation of AI infrastructure.
Data Sovereignty and Security in AI Training
As AI models deal with large and complex datasets, one of the questions being raised is how companies will look for ways to protect their proprietary data. Instead of training public models, companies will leverage proprietary engines that not only protect access to their data sources, but could help them gain a competitive edge in the marketplace. This takes data center infrastructure to a whole new level requiring even greater connectivity, agility, and scalability.
Verne's Approach to AI-Optimized Data Centers
At Verne, we understand the unique challenges and demands that AI models place on data centers. Our data centers are designed to reliably host, support and scale some of the most demanding AI models in the world, with facilities built to handle the high-density and high-performance needs of AI workloads.
As AI continues to reshape industries, we are staying one step ahead to meet these demands, adopting optimized designs that prioritize power, data center rack density, cooling, strength, and security. We know what powering progress looks like as we’ve been delivering high intensity compute for more than a decade.
Our AI-ready data centers offer high-density computing, sustainable power, and advanced cooling solutions to meet the demands of next-generation AI models.
Get in touch today to discover how our AI data center solutions can help your business.